Nivisha Parag, Rowen Govender, and Saadiya Bibi Ally, Regent Business School, South Africa
Contact: Nivisha.parag@regent.ac.za
Abstract
What is the message? Artificial intelligence (AI) is proving to be a transformative force in healthcare, promising improved diagnosis, treatment, and patient care. However, the application of AI in healthcare is not without challenges, especially in the context of cultural diversity. The authors propose a framework for AI adoption in culturally diverse settings, emphasizing the importance of ethical considerations, transparency, and cultural competence.
What is the evidence? The authors describe cases illustrating the complexities of AI in healthcare within culturally diverse contexts, and how their framework could be applied in culturally diverse regions of the world.
Timeline: Submitted: September 15, 2023; accepted after review November 26, 2023.
Cite as: Nivisha Parag, Rowen Govender, Saadiya Bibi Ally. 2023. Promoting Cultural Inclusivity in Healthcare Artificial Intelligence: A Framework for Ensuring Diversity. Health Management, Policy and Innovation (www.HMPI.org), Volume 8, Issue 3.
Introduction
Artificial intelligence (AI) is rapidly evolving into a powerful tool that is reshaping various industries, and healthcare is no exception. The integration of AI technologies in healthcare promises to revolutionize medical diagnosis, treatment, and patient care, with the aim of enhancing overall health outcomes. However, the successful implementation of AI in healthcare requires a nuanced understanding of the diverse cultural landscapes that influence healthcare practices and patient experiences worldwide.
Cultural diversity plays a pivotal role in healthcare, impacting patients’ beliefs, behaviors, and perceptions of health and illness. Cultural diversity in healthcare refers to the presence of individuals from different cultural, ethnic, linguistic, and socioeconomic backgrounds seeking healthcare services. These cultural factors significantly influence how individuals perceive health, interact with healthcare providers, and make healthcare decisions.1 AI and digital health technologies must adapt to these diverse cultural contexts to be effective and equitable.
This article explores the multifaceted role of AI in healthcare through the lens of cultural diversity. We examine the opportunities AI presents, such as personalized medicine and efficient healthcare delivery, alongside important ethical and cultural considerations. We will also assess the benefits and potential pitfalls of AI in healthcare within culturally diverse settings, emphasizing the need for responsible and inclusive AI implementation. The importance of acknowledging and including cultural diversity into AI implementation in healthcare is known, but guidance on its adoption in a manner that respects cultural diversity is limited. As such, we have developed a proposed framework for AI adoption in culturally diverse settings.
The following cases illustrate the complexities of AI in healthcare within culturally diverse contexts:
a. Diabetes Management in Indigenous Populations
Diabetes is a global health concern, affecting diverse populations. Indigenous communities, in particular, face unique challenges related to cultural practices, dietary habits, and access to healthcare.2 An AI-driven mobile application was developed to assist indigenous populations in managing diabetes.
The application incorporated culturally relevant dietary recommendations and incorporated traditional healing practices. AI algorithms analyzed blood glucose levels, physical activity, and dietary choices to provide personalized advice. The results were promising, with improved diabetes management and better adherence to treatment plans among indigenous individuals.
However, challenges emerged concerning data privacy and trust. Indigenous communities expressed concerns about the collection and use of their health data, requiring additional efforts to build trust and ensure data protection aligned with their cultural values.
b. Machine Translation in Multilingual Healthcare Settings
In culturally diverse healthcare settings with patients who speak multiple languages, communication barriers can hinder accurate diagnosis and treatment. An AI-driven machine translation system was implemented in a hospital to bridge these language gaps and provide quality care to patients.
The system allowed healthcare providers to communicate with patients in their preferred language, improving the patient-provider relationship and reducing misunderstandings. However, the accuracy of machine translation, especially for medical terminology, posed challenges. Misinterpretations of symptoms or treatment instructions occurred, highlighting the importance of human oversight and cultural competence.3
This article aims to review the role of AI in healthcare through the lens of cultural diversity.
AI in Healthcare: Cultural considerations and potential pitfalls
Diagnostic Advancements
AI has demonstrated remarkable capabilities in medical image analysis, aiding in the early and accurate diagnosis of various diseases. Machine learning algorithms excel at recognizing patterns and anomalies in medical images such as X-ray, MRI, and CT scans.4 These advancements have the potential to benefit individuals across diverse cultural backgrounds, ensuring timely and precise diagnoses. For instance, deep learning models have shown exceptional accuracy in detecting diabetic retinopathy, a condition that can lead to vision loss. This technology is vital for early intervention, especially in regions with limited access to specialized ophthalmologists. However, to harness the full potential of AI-driven diagnostics, it is imperative to ensure that the underlying datasets are representative of diverse populations to prevent biases that may disproportionately affect certain cultural groups. AI plays a crucial role in diagnostic and treatment planning, but its effectiveness can vary across different cultural groups. For example, diagnostic algorithms may need to consider variations in disease prevalence, symptom presentation, and genetic factors among different ethnicities. Culturally tailored diagnostic models can help ensure that AI-powered systems provide accurate and relevant recommendations to individuals from diverse backgrounds.
Personalized Medicine
One of the most promising applications of AI in healthcare is personalized medicine. By analyzing an individual’s genetic makeup, medical history, and lifestyle, AI can tailor treatment plans to the specific needs of the patient.5 This approach not only enhances treatment efficacy but also respects the cultural beliefs and preferences of patients.
For example, pharmacogenomics, which uses AI to predict an individual’s response to medication based on their genetic profile, can reduce adverse drug reactions and optimize treatment outcomes. In a culturally diverse healthcare setting, personalized medicine can accommodate variations in drug metabolism and efficacy among different populations.
However, the implementation of personalized medicine must be culturally sensitive. It is essential to consider cultural factors that may influence patients’ choices and adherence to treatment plans. Patient engagement and consent should be informed by an understanding of cultural norms and values.
Healthcare Delivery and Access
AI has the potential to revolutionize healthcare delivery by improving efficiency and accessibility. Telemedicine, powered by AI-driven chatbots and virtual health assistants, can bridge gaps in healthcare access, especially in remote or underserved areas. These technologies can provide medical advice, monitor patients’ conditions, and offer health education in multiple languages, accommodating cultural diversity.
Furthermore, AI can optimize hospital operations, from appointment scheduling to resource allocation. Predictive analytics can help healthcare providers anticipate patient needs and allocate resources effectively,6 ensuring that culturally diverse patient populations receive equitable care. In addition, equitable allocation of resources require diverse, representative data sets to build predictive algorithms.
Ethical Considerations in AI-Enabled Healthcare
Bias and Fairness
One of the central ethical challenges in AI-driven healthcare is addressing bias and ensuring fairness in AI algorithms. Biased algorithms can perpetuate healthcare disparities, particularly in culturally diverse populations. These biases can arise from biased training data or algorithmic design flaws, leading to inaccurate diagnoses or treatment recommendations.7
To mitigate bias, healthcare AI developers must prioritize diverse and representative datasets, which encompass various ethnic, cultural, and socioeconomic backgrounds. Additionally, continuous monitoring and auditing of AI systems are essential to identify and rectify bias that may emerge over time.7
Informed Consent
Informed consent is a fundamental ethical principle in healthcare, and its importance extends to AI applications. Patients must be adequately informed about how their data will be used in AI-driven healthcare solutions and should have the right to opt out if they choose.8 This is particularly crucial when dealing with culturally diverse populations, as cultural norms and expectations regarding data sharing may differ. Data security and privacy concerns are universal, but the cultural perspective on data sharing can vary significantly. In some cultures, there may be strong reservations about sharing health-related data, especially with technology companies or healthcare providers. AI-driven health systems should provide clear information about data usage and obtain culturally informed consent to address these concerns.
Cultural competence among healthcare providers and AI developers is essential to ensure that informed consent processes are culturally sensitive and respectful of patients’ beliefs and preferences. Patients from diverse backgrounds should feel comfortable participating in AI-enabled healthcare without fear of discrimination or exploitation. Safeguarding patient information is crucial, especially when dealing with sensitive cultural or health-related data. Striking a balance between data accessibility and security is an ongoing challenge.
Transparency and Accountability
Transparency in AI algorithms is critical for building trust among patients and healthcare providers. Patients have a right to understand how AI is being used in their healthcare, from diagnosis to treatment recommendations. Furthermore, establishing accountability mechanisms is essential to address any adverse outcomes or errors caused by AI.9
In culturally diverse healthcare settings, transparency is even more critical because patients may have different expectations and understandings of AI’s role in their care. Clear communication and education about AI’s capabilities and limitations can help foster trust and facilitate culturally competent care.
A framework for AI adoption in culturally diverse settings
Realizing the full potential of AI and digital health solutions in culturally diverse healthcare settings necessitates a multifaceted approach that prioritizes cultural competence, fairness, and sensitivity throughout the entire lifecycle of these technologies, from design and deployment to evaluation and ongoing refinement. A proposed framework for the adoption of AI in culturally diverse settings would give consideration to each item shown in the following diagram:
Figure 1: A Framework for AI Adoption in Culturally Diverse Settings
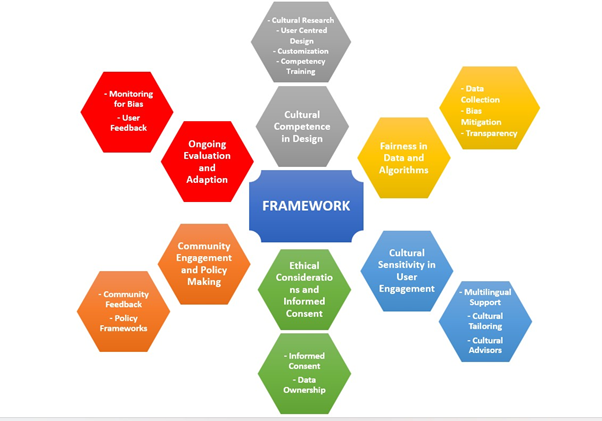
The next section explores each item in the framework in greater detail:
1. Cultural Competence in Design:
Cultural competence begins at the design phase of AI and digital health solutions. Developers and designers must ensure that their technologies are inclusive and respectful of diverse cultural backgrounds. This involves:
Cultural Research: Conduct extensive research into the target cultural populations to understand their health beliefs, practices, and preferences. This knowledge informs the design choices, such as the use of culturally appropriate imagery, language, and symbols.
User-Centered Design: Apply user-centered design principles to create interfaces and user experiences that are intuitive and engaging for individuals from various cultural backgrounds. This may involve conducting usability testing with diverse user groups to identify and address cultural usability issues. Culturally competent design fosters trust and enhances user engagement.
Customization: Allow for customization or personalization of the user experience, where individuals can tailor the interface or content to align with their cultural preferences. This fosters a sense of ownership and comfort among users.
Cultural Competency Training: Offer training to healthcare professionals and AI system operators on cultural competency and sensitivity. This ensures that they can effectively use and support these technologies in diverse healthcare settings.
Consideration must be given to the cultural beliefs, norms, and practices of the target population when designing algorithms and user interfaces. Collaboration with healthcare professionals from diverse backgrounds and the involvement of cultural advisors can facilitate this process.
2. Fairness in Data and Algorithms:
Ensuring fairness in AI-driven healthcare solutions is critical to avoid perpetuating biases and health disparities. Key considerations include:
Data Collection: Ensure that the training datasets used for AI algorithms are diverse and representative of the population. Biased datasets can lead to biased algorithms that may not work equally well for all cultural groups. Datasets should encompass various ethnic, cultural, and socioeconomic groups to ensure that AI-driven healthcare solutions are equitable and accurate for all patients. It is essential to continuously monitor and assess AI systems for potential bias, as these technologies can inadvertently exacerbate disparities if not carefully designed and tested for fairness across diverse populations.
Bias Mitigation: Implement bias mitigation techniques, such as re-sampling underrepresented groups or adjusting algorithmic weights, to ensure equitable performance across diverse populations. Studies have revealed that AI algorithms trained predominantly on male datasets may perform alarmingly poorly when diagnosing medical conditions in women. This discrepancy can result in gender-based disparities in healthcare outcomes, with some research indicating that certain algorithms exhibit an error rate of up to 47.3% in identifying heart disease in women compared to just 3.9% in men.10 Furthermore, biased data can perpetuate racial or ethnic disparities in disease diagnosis and treatment recommendations, with some investigations showcasing that certain AI systems exhibit significantly lower accuracy rates when diagnosing skin conditions in darker-skinned individuals compared to lighter-skinned individuals, with an error rate disparity as high as 12.3%.10
Unintended bias in algorithms is another concern, with potential to worsen social and healthcare disparities. The decisions made by AI systems reflect the input data they receive, so it’s crucial that this data accurately represents patient demographics. Additionally, gathering data from minority communities can sometimes result in medical discrimination. For instance, HIV prevalence in minority communities can lead to the discriminatory use of HIV status against patients.11
Moreover, variations in clinical systems used to collect data can introduce biases. For example, radiographic systems, including their outcomes and resolution, differ among providers. Additionally, clinician practices, such as patient positioning for radiography, can significantly influence the data, making comparisons challenging.12
However, these biases can be mitigated through careful implementation and a systematic approach to collecting representative data.
Transparency: Provide transparency in algorithmic decision-making, so users and healthcare providers can understand how AI-generated recommendations are made. This transparency helps build trust.
3. Cultural Sensitivity in User Engagement:
Effective user engagement is essential for the adoption and success of AI and digital health solutions. To engage diverse cultural groups the following needs consideration:
Multilingual Support: Offer multilingual support for user interfaces and communication. This ensures that individuals who speak languages other than the dominant language in the healthcare system can access and use the technology.
Cultural Tailoring: Customize content and recommendations based on cultural preferences and health beliefs. For instance, dietary recommendations or health education materials can be tailored to align with cultural norms.
Cultural Advisors: Collaborate with cultural advisors or community leaders to ensure that the technology aligns with cultural values and practices. Their insights can guide content and engagement strategies.
4. Ethical Considerations and Informed Consent:
Respecting cultural diversity also means addressing ethical considerations related to data privacy and informed consent:
Informed Consent: Ensure that the informed consent process is culturally sensitive. This may involve providing information in multiple languages, using culturally appropriate communication channels, and respecting cultural norms regarding decision-making.
Data Ownership: Clearly define data ownership and usage rights, considering cultural perspectives on data sharing and control. This fosters trust and empowers individuals to make informed decisions about their data.
Guidelines and accountability: Regulatory bodies and healthcare organizations should establish clear ethical guidelines for the development and deployment of AI in healthcare. These guidelines should address issues related to bias, transparency, informed consent, and accountability. Oversight mechanisms should be put in place to monitor AI systems and ensure compliance with ethical standards.
5. Community Engagement and Policymaking:
Engaging with culturally diverse communities and involving them in policymaking and decision-making processes is vital:
Community Feedback: Actively seek feedback from diverse user groups to continuously improve and refine AI and digital health solutions. Communities can provide valuable insights and identify issues that may not be apparent to developers.
Policy Frameworks: Develop and implement policies and regulations that promote cultural competence, fairness, and sensitivity in healthcare technologies. These policies can set standards for inclusivity and equity in digital health.
Ongoing Evaluation and Adaptation:
The journey does not end with deployment; continuous evaluation and adaptation are essential:
Monitoring for Bias: Continuously monitor AI systems for bias and disparities, with a particular focus on how they affect different cultural groups. Addressing biases promptly is crucial.
User Feedback: Solicit and act on user feedback to refine and adapt the technology over time. Users’ needs and cultural contexts may evolve.
Sample framework deployment
In each of the scenarios described below, the proposed framework can ensure that AI-driven healthcare solutions are culturally competent, sensitive, and fair, addressing the unique cultural and healthcare challenges of the regions while promoting inclusivity and equity:
South Africa, as just one example, boasts a rich diversity of cultural and ethnic groups, with eleven official languages (twelve when sign language is included), and each with their own unique traditional healing systems. These systems are deeply rooted in indigenous knowledge, spirituality, and the interconnectedness of individuals with their environment. All of these elements require that AI technologies respect and integrate these cultural aspects as being essential for the successful adoption, acceptance, and effectiveness of such solutions. AI-powered language translation tools are invaluable in overcoming language barriers in healthcare settings with diverse patient populations. These tools can help healthcare providers communicate effectively with patients who speak different languages, ensuring that patients understand their diagnoses and treatment options. However, the accuracy of these tools and their ability to handle medical terminology in various languages must be continuously improved to avoid miscommunication.
By deploying the proposed framework to the South African landscape, the following important considerations would be addressed: developers of AI-powered language translation tools can conduct cultural research to understand the linguistic diversity and health beliefs of different cultural groups. This knowledge can inform the design of user interfaces and content, incorporating culturally appropriate imagery and multilingual support. Customization options should be offered, allowing users to tailor their experience based on their cultural preferences. It is also essential to ensure that AI algorithms are trained on diverse and representative datasets that encompass the various cultural and linguistic groups. Bias mitigation techniques should be in place to address disparities in performance across diverse populations. Cultural competency training for healthcare professionals and AI system operators should be implemented to ensure that they can effectively use and support these technologies in diverse healthcare settings. Collaboration with traditional healers and community leaders would ensure that the technology aligns with cultural values and practices. Community feedback would be actively sought to refine AI and digital health solutions.
Similarly, in Japan, a country renowned for its rapidly aging population, healthcare systems face the unique challenge of providing culturally sensitive care to elderly individuals. AI technologies are being thoughtfully adapted to address these concerns, ranging from AI-driven robotic companions that cater to specific cultural norms and preferences to the development of AI-powered monitoring systems that respect the dignity and privacy of elderly patients.
The framework would ensure that user-centered design principles are applied, with usability testing involving diverse user groups. This ensures that interfaces are intuitive and engaging for individuals from these various cultural backgrounds. Content and recommendations can be culturally tailored to align with the health beliefs and preferences of the aging population.
In the United States, a nation characterized by its rich cultural diversity, healthcare disparities among immigrant populations are a pressing concern. AI applications are emerging as crucial tools in reducing these disparities by facilitating better communication and care delivery in multilingual and multicultural healthcare settings. These applications include AI-driven language translation tools, culturally tailored health education platforms, and AI-assisted diagnostic tools that account for the diverse backgrounds and perspectives of patients.
Applying elements of the framework in this setting can address bias mitigation techniques to ensure that AI-driven language translation tools and diagnostic tools do not perpetuate healthcare disparities among immigrant populations. Continuous monitoring for bias and disparities in AI systems, with a focus on how they affect different cultural groups, should be carried out in all scenarios. User feedback should be solicited and acted upon to refine and adapt the technology over time, considering evolving user needs and cultural contexts.
In all case scenarios, the informed consent process should be culturally sensitive, providing information in multiple languages and respecting cultural norms regarding decision-making.
By applying the framework elements to the described case scenarios, AI and digital health solutions can become more culturally competent, respectful, and inclusive, ultimately improving healthcare outcomes for diverse populations in South Africa, Japan, the United States and many other countries. This framework provides a robust, holistic approach to AI adoption in culturally diverse healthcare settings.
Conclusion
Artificial intelligence holds immense promise in revolutionizing healthcare by enhancing diagnosis, treatment, and healthcare delivery. However, in the context of cultural diversity, it presents both opportunities and challenges. Understanding and respecting cultural norms and values is essential to harness the full potential of AI in healthcare while avoiding biases and disparities.
This article has explored the multifaceted role of AI in healthcare within culturally diverse settings, emphasizing the importance of ethical considerations, transparency, and cultural competence. Empirical case studies have illustrated how AI can benefit diverse populations while highlighting the need for ongoing monitoring and adaptation to ensure culturally sensitive care.
As AI continues to advance in healthcare, stakeholders must prioritize inclusivity and cultural diversity to ensure that these transformative technologies benefit all individuals, regardless of their cultural background. The successful integration of AI and digital health in culturally diverse settings necessitates a nuanced understanding of the complex interplay between technology and cultural diversity. Achieving the full potential of AI and digital health in culturally diverse contexts requires a holistic approach that integrates cultural competence, fairness, and sensitivity into every aspect of technology development and deployment. By prioritizing these principles, developers, healthcare organizations, and policymakers can create healthcare technologies that are truly inclusive, equitable, and effective for all individuals, regardless of their cultural backgrounds.
References
- Hall WJ, Chapman MV, Lee KM, Merino YM, Thomas TW, Payne BK, Eng E, Day SH, Coyne-Beasley T. Implicit Racial/Ethnic Bias Among Health Care Professionals and Its Influence on Health Care Outcomes: A Systematic Review. Am J Public Health. 2015 Dec;105(12):e60-76. doi: 10.2105/AJPH.2015.302903. Epub 2015 Oct 15. PMID: 26469668; PMCID: PMC4638275.
- Pham Q, Gamble A, Hearn J, Cafazzo JA. The Need for Ethnoracial Equity in Artificial Intelligence for Diabetes Management: Review and Recommendations. J Med Internet Res. 2021 Feb 10;23(2):e22320. doi: 10.2196/22320. PMID: 33565982; PMCID: PMC7904401.
- Greenhalgh T, Wherton J, Papoutsi C, Lynch J, Hughes G, A’Court C, Hinder S, Fahy N, Procter R, Shaw S. Beyond Adoption: A New Framework for Theorizing and Evaluating Nonadoption, Abandonment, and Challenges to the Scale-Up, Spread, and Sustainability of Health and Care Technologies. J Med Internet Res. 2017 Nov 1;19(11):e367. doi: 10.2196/jmir.8775. PMID: 29092808; PMCID: PMC5688245.
- Tang X. The role of artificial intelligence in medical imaging research. BJR Open. 2019 Nov 28;2(1):20190031. doi: 10.1259/bjro.20190031. PMID: 33178962; PMCID: PMC7594889.
- Lupton D. Data selves: More-than-human perspectives. In Data selves, 1-24. Polity; 2019.
- Bajwa J, Munir U, Nori A, Williams B. Artificial intelligence in healthcare: transforming the practice of medicine. Future Healthc J. 2021 Jul;8(2):e188-e194. doi: 10.7861/fhj.2021-0095. PMID: 34286183; PMCID: PMC8285156.
- Ueda, D., Kakinuma, T., Fujita, S. et al. Fairness of artificial intelligence in healthcare: review and recommendations. Jpn J Radiol (2023). https://doi.org/10.1007/s11604-023-01474-3
- Kahn JM, Parekh A. Ethical considerations in machine learning for health care. JAMA. 2020;323(18):1847-1848.
- Naik N, Hameed BM, Shetty DK, Swain D, Shah M, Paul R, Aggarwal K, Ibrahim S, Patil V, Smriti K, Shetty S. Legal and ethical consideration in artificial intelligence in healthcare: who takes responsibility? Front Surg. 2022;9:266.
- Celi, L. A., Cellini, J., Charpignon, M. L., Dee, E. C., Dernoncourt, F., Eber, R., Mitchell, W. G., Moukheiber, L., Schirmer, J., Situ, J., Paguio, J., Park, J., Wawira, J. G., Yao, S., & for MIT Critical Data (2022). Sources of bias in artificial intelligence that perpetuate healthcare disparities-A global review. PLOS digital health, 1(3), e0000022. https://doi.org/10.1371/journal.pdig.0000022
- Nordling L (September 2019). “A fairer way forward for AI in health care”. Nature. 573 (7775): S103–S105. Bibcode:2019Natur.573S.103N. doi:10.1038/d41586-019-02872-2
- Pumplun L, Fecho M, Wahl N, Peters F, Buxmann P (October 2021). “Adoption of Machine Learning Systems for Medical Diagnostics in Clinics: Qualitative Interview Study”. Journal of Medical Internet Research. 23 (10): e29301. doi:10.2196/29301. PMC 8556641. PMID 34652275. S2CID 238990562.